Inpainting, the art of seamlessly repairing small imperfections in images, is a valuable technique that can work wonders for enhancing your visual content. In this comprehensive guide, we’ll walk you through step-by-step examples of how to harness the power of Inpainting in Stable Diffusion.
Whether you’re a digital artist, a photo enthusiast, or simply someone with an eye for detail, inpainting is a skill that can take your image editing prowess to new heights. So, let’s dive in and uncover the art of perfecting your images with it.
What does Inpainting mean?
Inpainting is a technique used to restore or repair images by intelligently filling in missing or damaged parts. This process is commonly employed in image editing and restoration to rectify imperfections or remove undesired objects or blemishes from an image. The objective is to make the final image appear smooth and unaltered as if the missing or problematic elements were never there.
Inpainting finds applications in diverse fields, including film restoration, photo enhancement, and the creation of digital art. It’s a versatile tool for improving and enhancing images in a natural and seamless manner.
How does Inpainting work in Stable Diffusion?
Inpainting in Stable Diffusion utilizes the principles of heat diffusion to repair missing or damaged parts within an image. This technique involves applying a heat diffusion process to the pixels surrounding the affected area, resulting in a cohesive and natural patch that seamlessly integrates with the rest of the image.
Here’s a detailed breakdown of how Inpainting works in Stable Diffusion:
- Encoder: The process begins with the input image being passed through the encoder portion of the model. The encoder’s role is to compress the input image into a lower-dimensional latent representation. This representation captures essential features and information from the original image, effectively summarizing its content.
- Masking: To specify the region of the image that needs inpainting, a mask is applied. This mask identifies the area where pixels are missing or should be replaced. The pixels within this masked region are typically removed, creating a gap in the image.
- Decoder: The incomplete image, with the masked area, is then fed into the decoder part of the model. The decoder’s task is to reconstruct the original image from the latent features obtained in the encoding step. However, it faces a challenge because the masked region contains missing information.
- Inpainting: The key aspect of inpainting is how the model handles the missing information in the masked region. The decoder generates new content to fill in this area while ensuring that the generated content matches the surrounding image features and context. This process aims to make the inpainted section appear as though it was always a seamless part of the original image.
- Generative Capabilities: The generator component of the decoder has learned a robust understanding of image composition through training on extensive datasets. This enables it to produce content that not only matches the surrounding context but also looks visually coherent and realistic.
- Variability: To ensure diverse and interesting results, the model utilizes noise during the generation process. By sampling repeatedly with noise, it can produce multiple variations of the inpainted section. This allows for a range of options to choose from, ensuring that the process is not overly deterministic.
- Textual Guidance: In addition to the automatic inpainting process, users can provide text prompts to guide it towards a specific semantic meaning. This means that textual descriptions can influence the content generated to fill in the masked region, helping to achieve inpainting results that align with desired artistic or contextual intentions.
Inpainting in Stable Diffusion relies on an autoencoder architecture with strong generative capabilities. It uses the encoder to compress the input image, applies a mask to identify the region to be inpainted, and then uses the decoder to generate content that seamlessly fills in the missing or corrupted part of the image while maintaining visual coherence.
The model’s generative power, noise sampling, and textual guidance options contribute to the versatility and realism of the inpainting process.
How is It different from other Inpainting Techniques?
Stable Diffusion Inpainting distinguishes itself from other image inpainting methods due to its exceptional stability and ability to produce smooth results.
Many alternative techniques often suffer from issues like slow processing speeds, instability, or the generation of noticeable artifacts that can detract from the naturalness of image repairs. In contrast, Stable Diffusion Inpainting shines in handling images with intricate structures, such as textures, edges, and abrupt transitions.
Inpainting methods based on diffusion operate by transferring information from the neighboring regions of an image into the sections that are either missing or damaged. This methodology offers several merits:
- It can generate outcomes that are smooth and visually coherent, rendering it applicable to a diverse array of uses.
- Implementing this technique is relatively straightforward, and it boasts computational efficiency in contrast to alternative methods.
Step-by-Step Inpainting in Stable Diffusion
Let’s learn how to do Inpainting in Stable Diffusion with each step in detail:
Step 1: Generating the Base Image
Begin by creating an original image using the txt2img feature in Stable Diffusion. Identify specific issues within the image that you wish to address, such as unnatural facial features or the need for a different object, like a villa, in the picture.
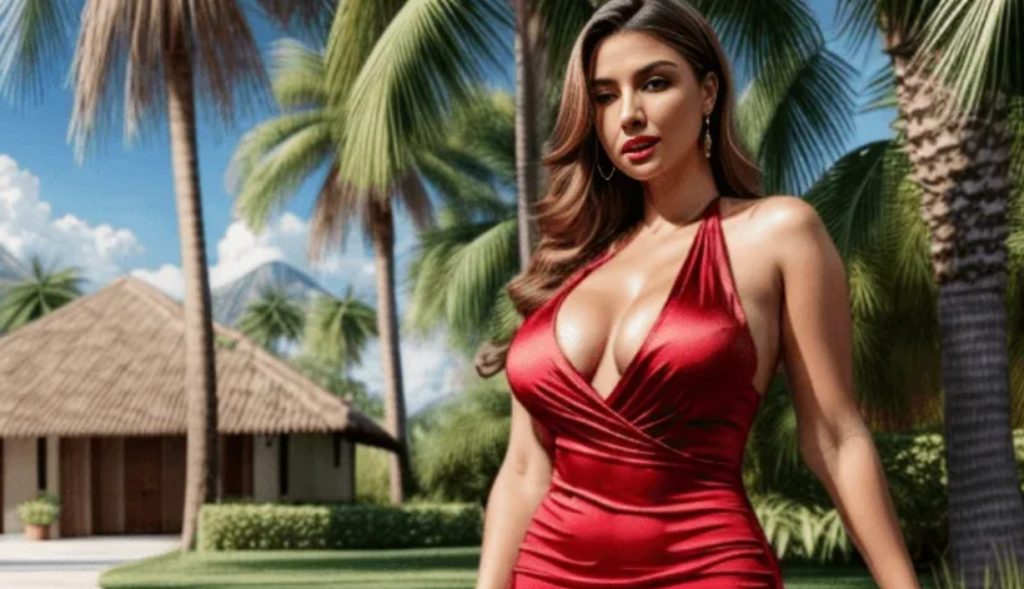
Step 2: Initiating the Inpainting Process
Click the “Send to inpaint” button beneath the generated image to start the inpainting process. For images not initially created using txt2img, navigate to the img2img tab, select ‘inpaint,’ and upload your image to begin the procedure.

Step 3: Creating an Inpaint Mask
Utilize the AUTOMATIC1111 GUI to create an inpainting mask. Focus on the regions you want to inpaint, such as the face. Use the paintbrush tool to create a mask indicating the areas Stable Diffusion should regenerate.
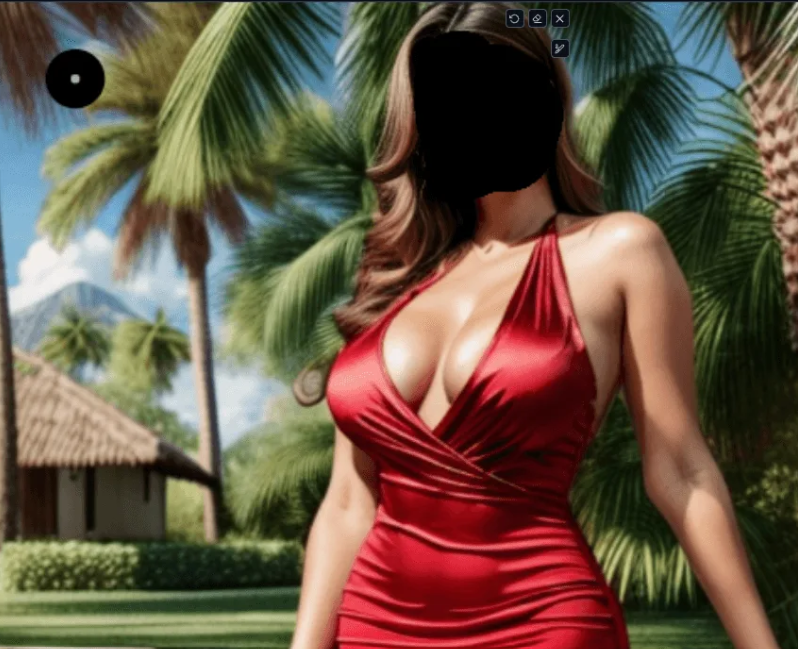
Step 4: Adjusting Inpainting Settings
Fine-tune various settings for optimal results:
- Prompt: Modify the original prompt to address specific changes you desire. This ensures focused inpainting.
- Image Size: Adjust the image size to match the original image dimensions for consistency.
- Face Restoration: Enable “Restore faces” if inpainting facial features.
- Masked Content: Choose “original” for guidance from the original content’s color and shape.
- Denoising Strength: Adjust to control the extent of changes compared to the original image.
- Batch Size: Generate multiple unique images by setting the seed to -1.
- CFG Scale: Balance prompt influence; a value of 7 usually strikes a good balance.
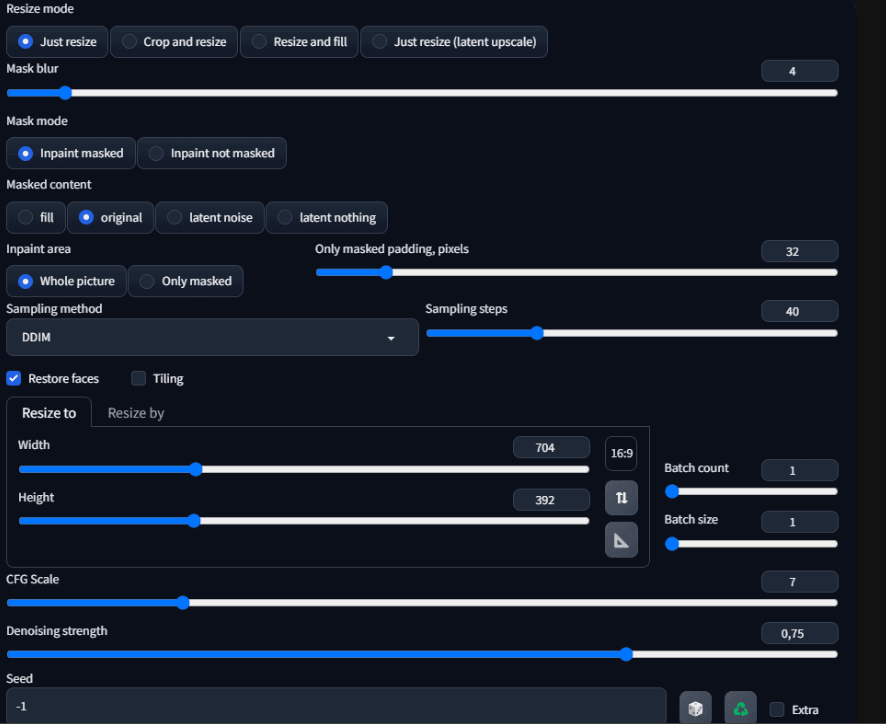
Step 5: Reviewing Inpainting Results
Examine the inpainted images generated using the configured settings. Assess the effectiveness of inpainting in fixing defects and enhancing the overall image quality. Notice the natural and significant improvements, especially in facial features.
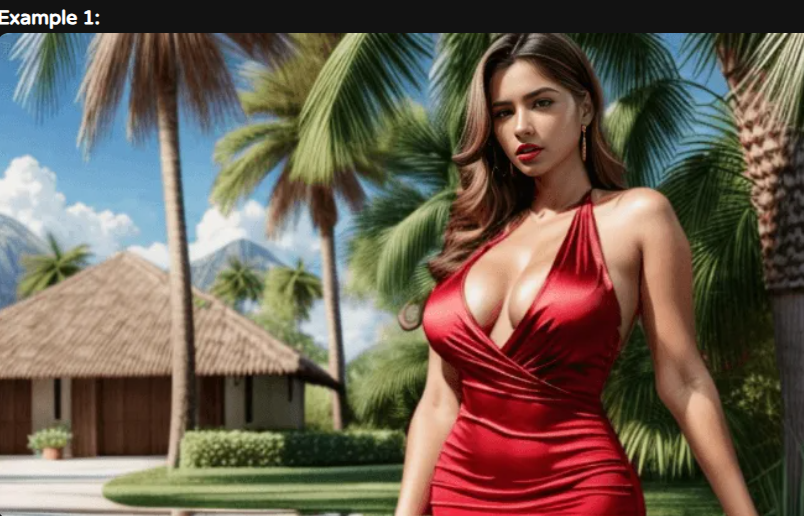
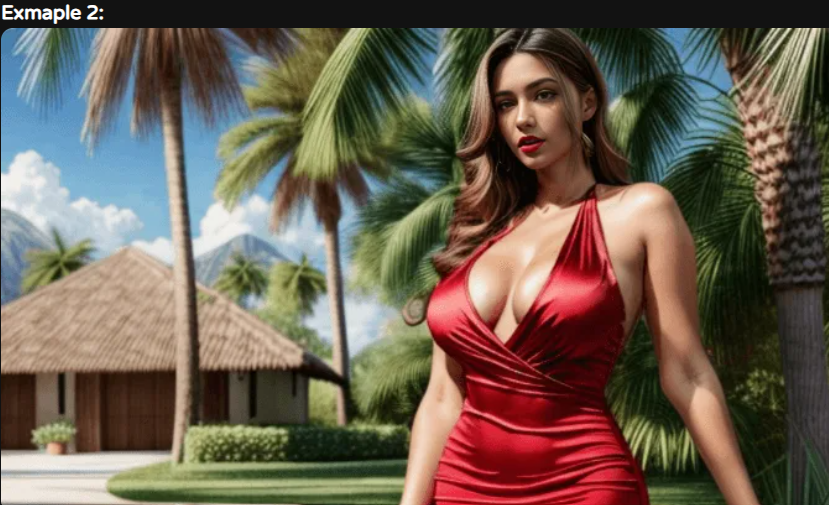
Step 6: Inpainting New Objects
Explore the creative potential of inpainting by adding new objects to an image. Use the brush tool to inpaint a mask, specifying the area where the new object, such as a villa, should be placed.

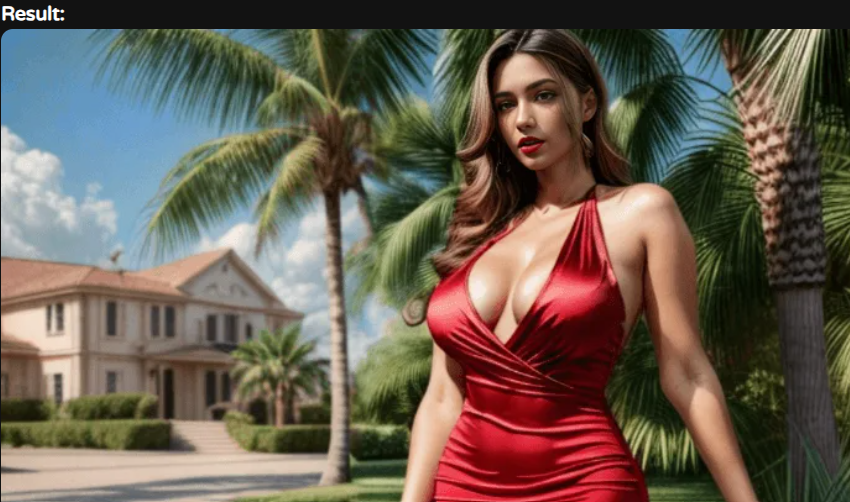
By following these steps, you can leverage Stable Diffusion’s inpainting capabilities to address specific image issues, enhance facial features, and even introduce new elements into your compositions, resulting in impressive and natural-looking edits.
Inpainting Tips for Beginners
Inpainting can indeed be a valuable tool for image editing, but it does require some patience and skill, especially for beginners. Here’s an elaborate breakdown of the tips mentioned for beginners looking to achieve successful results:
- Work on Small Areas
- Focusing on small areas at a time allows for better control and attention to detail.
- It’s advisable to start with minor imperfections or smaller missing elements in your image.
- Masked Content Settings
- Keeping the masked content at “Original” often yields more natural and contextually coherent results.
- Adjusting the denoising strength can help refine the inpainting and control the level of detail in the result.
- Experiment with Masked Content Options
- Different masked content options (e.g., “Original,” “Random,” or “Custom”) are available in inpainting tools.
- Experimenting with these options helps you find the most suitable one for your specific inpainting task.
Limitations You Should Know
Here are some key limitations you should be aware of when using Inpainting in Stable Diffusion:
- Imperfect Realism: While inpainting in Stable Diffusion can produce impressive results, it’s important to note that perfect realism cannot always be achieved. In certain cases, the edited portions of an image may still exhibit subtle discrepancies that could be discerned upon close examination.
- Challenges with Complex Tasks: The model may face difficulties when dealing with more complex tasks, especially those involving patterned or textured surfaces. In such scenarios, the inpainted areas might appear mismatched or unnatural, potentially detracting from the overall quality of the edited image.
- Language Dependency: The inpainting model was primarily built and trained using English captions. This limitation can make it challenging to generate images based on prompts in other languages. When attempting to do so, the results may not be as accurate or satisfactory as those generated from English prompts.
- Data Security Concerns: When using AI-generated images, there’s a security risk involved. Sensitive data could potentially be embedded in these images, and if they fall into the wrong hands, it could lead to privacy and security issues. Users should exercise caution when sharing or distributing AI-generated content.
- Potential for Misleading Content: Inpainting tools, including those in Stable Diffusion, have the ability to seamlessly add or replace elements within an image, creating a visually convincing result. However, this can also be a limitation as it may lead to the creation of misleading or deceptive content that differs significantly from reality. Users should be mindful of ethical considerations when using such tools.
While inpainting offers powerful image editing capabilities, it is not without its limitations. Users should be aware of these constraints, including the potential for imperfect realism, challenges with complex tasks, language dependencies, data security risks, and the potential for misleading content. Understanding these limitations can help users make informed decisions when employing inpainting tools and ensure they use them responsibly and ethically.
Real-World Applications
Inpainting is an incredibly versatile technique with a wide range of real-world applications, particularly when implemented using advanced methods like Stable Diffusion. Here are several practical examples of how inpainting can be applied effectively:
- Object Removal: It can be used to seamlessly remove unwanted objects or individuals from the background of a photo, resulting in a cleaner and more aesthetically pleasing image.
- Photo Restoration: It is an invaluable tool for restoring old or damaged photographs by filling in missing or corrupted sections, breathing new life into cherished memories.
- Background Replacement: It allows you to mask out the existing background and replace it with something more suitable or visually interesting, transforming the overall atmosphere of an image.
- Watermark Removal: You can use inpainting to cover up distracting text, logos, or other overlays, making images more professional or suitable for various purposes.
- Adding Context: It can be employed to expand the context of a focused portrait by seamlessly integrating a relevant background scene, enhancing the storytelling aspect of the image.
- Compositing: By sequentially inpainting elements from multiple images, you can create composite images, blending different visual elements seamlessly.
- Iterative Editing: It can facilitate progressive image modifications by allowing for multiple rounds of inpainting, enabling fine-tuning and adjustments over time.
- Image Expansion: Extend the canvas size of an image beyond its original boundaries by inpainting new areas, enabling creative compositions and layouts.
- Detail Enhancement: It can be used to enhance specific details within an image, such as textures, patterns, or high-resolution elements, resulting in a more visually appealing outcome.
- Photo to Art: Employ inpainting with style transfer techniques to transform ordinary photos into various artistic styles, offering a unique and creative approach to image processing.
- Art Refinement: Targeted inpainting enables adjustments to proportions, lighting, colors, and details within artwork, allowing for fine-tuning and artistic refinement.
- Text Removal: Eliminate unwanted text, captions, or speech bubbles from comic panels or images, preserving the visual integrity of the content.
The versatility of inpainting, particularly when harnessed with advanced methods like Stable Diffusion, enables both major and subtle post-generation image edits. This adaptability makes it a powerful tool for realizing creative visions across a spectrum of real-world applications, from image restoration to artistic expression and beyond.
Now you can move to learn more about Textual Inversion in Stable Diffusion here.
Takeaways
Inpainting, especially when combined with cutting-edge techniques like Stable Diffusion, emerges as an incredibly versatile tool capable of facilitating a wide range of post-generation image modifications. Its adaptability empowers creators to bring their creative visions to life in various real-world scenarios, spanning from image restoration to artistic expression and beyond.
Whether it’s seamlessly restoring damaged photographs, generating imaginative artworks, or making subtle yet impactful edits, inpainting stands as a potent resource for unlocking the full potential of visual content.